4 Types of Data Analytics Every Analyst Should Know-Descriptive, Diagnostic, Predictive, Prescriptive
At its core, data analytics is about answering questions and making decisions. And just as there are different types of questions, there are also different types of data analytics depending on what you’re hoping to accomplish. Four primary types of data analytics:
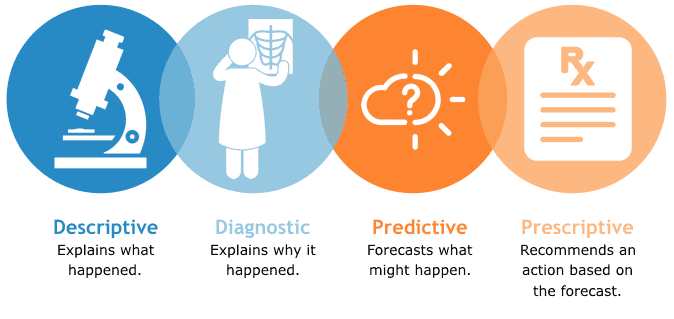
Descriptive analytics
What happened?
Descriptive analytics juggles raw data from multiple data sources to give valuable insights into the past. However, these findings simply signal that something is wrong or right, without explaining why?
For example, Data analysts are working with an e-commerce marketing team to review sales data to identify the sales trends and patterns, you will see an increase or decrease in sales from last year, specifically in what region and by what percentage.
Diagnostic analytics
Why did something happen?
Like descriptive analytics, diagnostic analytics also focus on the past. However, these types of analyses look for cause and effect to illustrate why something happened. The objective is to compare past occurrences to determine causes. Of course, this is seldom a black-and-white answer but instead provided in the context of probability, likelihood, or a distributed outcome.
Diagnostic analytics can guide by helping to:
- Identify outliers
- Isolate patterns
- Uncover relationships
Back to our marketing example, now that you are aware that there is a drop in sales, you can identify the reason why there is a sudden drop in sales? This may need additional examination for that might need to look at additional data points like website traffic, marketing budgets, and product inventory availability and make a correlation to uncover relationships. Using more complex analytics, analysts may employ probability theory, regression analysis, or time series to isolate cause and effect relationships.
Predictive analytics
What is LIKELY to happen?
Predictive analytics tells what is likely to happen. It uses the findings of descriptive and diagnostic analytics to detect clusters and exceptions and predict future trends, which makes it a valuable tool for forecasting. It brings many advantages like sophisticated analysis based on machine learning or deep learning and a proactive approach that predictions enable.
It is important to keep in mind, however, that no analytics will be able to tell you exactly what WILL happen in the future. Predictive analytics put in perspective what MIGHT happen, providing respective probabilities of likelihoods given the variables that are being looked at.
Now that we know the reason for the drop in sales. Predictive analytics helps to find what would be the expected sale in the next month, quarter, year, etc. The goal is to determine a trend, correlation, causation, or probability for the next purchase.
Prescriptive analytics
What action should be taken?
The purpose of prescriptive analytics is to literally prescribe what action to take to eliminate a future problem or take full advantage of a promising trend. It uses advanced tools and technologies, like machine learning, business rules, and algorithms, which makes it sophisticated to implement and manage. Besides, this state-of-the-art type of data analytics requires not only historical internal data but also external information due to the nature of the algorithms on its base.
As part of prescriptive analytics, if sales are falling then you can make timely decisions such as to cut prices, market more, or discontinue the product. If an item is selling off the shelves, you can be sure to stock inventory accurately across channels.
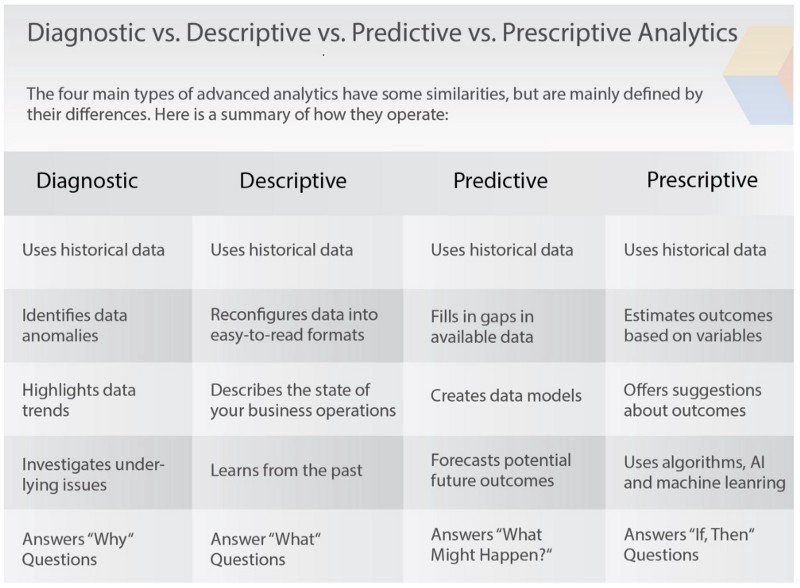
Data analysts can tailor their work and solution to fit the scenario. For instance, if a manufacturer is plagued with delays and unplanned stoppages, a diagnostic analytics approach could help identify what exactly is causing these delays. From there, other forms of analysis can be used for fixing these issues.
Example,
Descriptive analytics shows that whenever a deadly pandemic hits the human race, there is an exponential increase in the admission of patients to the hospital.
Diagnostic analytics would explore the data and make correlations. For instance, it may help you determine that all of the patients’ symptoms — high fever, dry cough, and fatigue — point to the same infectious agent.
Predictive analytics may forecast a surge in patients admitted to the hospital in the next several weeks or months. Based on patterns in the data, the illness is spreading at a rapid rate.
Now that you know the illness is spreading, prescriptive analytics may suggest that you increase the number of staff/medicine supplies on hand to adequately treat the influx of patients.
If you like what we do and want to know more about our community 👥 then please consider sharing, following, and joining it. It is completely FREE.
Also, don’t forget to show your love ❤️ by clapping 👏 for this article and let us know your views 💬 in the comment.
Join here: https://blogs.colearninglounge.com/join-us