Statistics 101: A No BS Introduction for Dummies
An ideal beginning would have been “Statistics is a science ….”. But, since this is a no BS introduction we have avoided lame definitions and jargon. To share the easiest introduction let us begin with something that all of us can relate to, ‘Sports’.
Pick a sport of your choice and a team in that sport you closely follow. Say, your team is competing in a tournament and is having a rough patch having lost two of the last three games that they have played which makes you as a fan anxious 😨. But, when you look at the overall performance in the tournament so far, you observe that they have won nine out of the twelve games played in the tournament so far.
Now, let’s take a step back and build some intuition around this. When we look at the performance based on the past two games, the success rate of the team comes out to be ~33%, but when we look at the overall performance in the tournament so far, the success rate comes out to be 75%. This is one of the primary applications of statistics, it helps us look at the bigger picture by ‘summarizing’ events into a single number.
Further, say, there is an upcoming match against a strong opponent and you need to know the chances of your team winning the match. You turn the pages of history to look at your team’s past head-to-head performance against the very opponent. You find out that your team has won 70% of the past head-to-head games. This statistic gets you all excited about the chances of your team in the upcoming game. But, then a realization hits you that your team has lost two of the last three matches and the opponent is on a winning streak as a result of which your team lacks momentum. After you factor in your recent realization, you see that the chances of your team winning have dropped, but they still do have slightly higher chances of winning.
This is another aspect of statistics, they help you build an estimation for the future based on the observations and intuitions of the past. Now, does that mean your team will win the upcoming match given your estimates? Well, maybe, maybe not.
“Statistics do not give a single right answer or a single right way of looking at things, what it does is it helps condense useful information in an easily understandable manner.”
To do good statistics, you need good data. Data is merely the set of observations. Data is the raw material required to do statistics. The better the raw material, the better the final product. In the case of statistics, the better the data, the more reliable the statistic.
So, with that said, let’s just understand the most general forms in which data normally comes.
TYPES OF DATA(STATISTICAL VARIABLES)
Continuous
It is a kind of data that has an infinite number of values in a given range. For example, Income is a continuous variable with a wide range whereas something like the temperature has a very narrow range but the number of values that these variables can possibly have is very high.
Categorical
It takes a small finite number of distinct values. There are two types of categorical variables based on whether the categories are ordered or not.
For example, a categorical variable like Education Level that has categories like ‘Less than high school’, ‘High school graduate’, ‘College graduate’,’ Masters degree and higher’ are ordered categories i.e. they aren’t completely independent of each other. Such a categorical variable is known as an ‘Ordinal Variable’.
The categorical variables such as Gender where categories like Male, Female, and Others are totally independent of each other are called ‘Nominal Variables’.
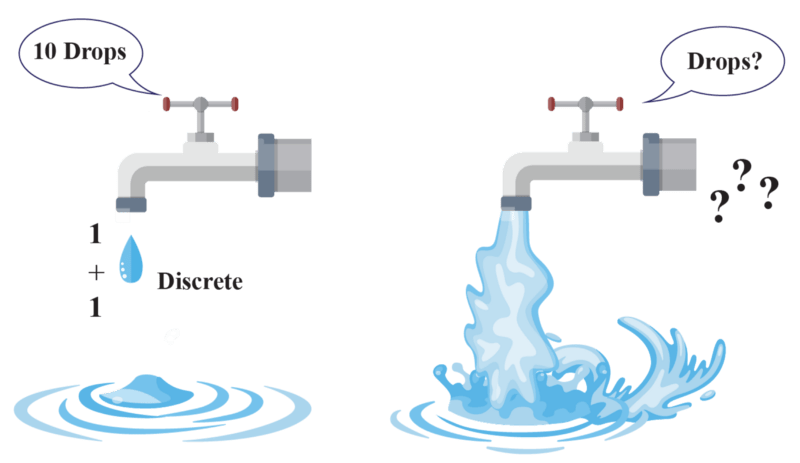
Now we understand the data and its types. Let us shift our focus to the crux of this piece. How do we build on this data and what are the primary ways in which we can get something out of the data. Almost all statistics fall under these two broad functions: Summarization, and Estimation or more technically, Descriptive Statistics and Inferential Statistics.
DESCRIPTIVE vs INFERENTIAL STATISTICS
Summarization
Summarization deals with methods used to summarize or describe the observations, and it falls under the category of Descriptive Statistics. In the first part of the sports example, we essentially summarized the performance of the team based on the past three games and then based on the performance in the tournament so far and we looked at the success rates for both these cases. The success rate here is our ‘Statistic’ since it helps us summarize our observations from the past. There are a number of methods that are used to describe data in different ways depending upon what kind of answers you need from the data or what outcome you seek from the study.
Descriptive Statistics is also used to build insights using data over a time interval where a statistic is calculated for a number of time intervals and then compared across intervals. For example, economic indicators like the Unemployment rate or % GDP growth are meant to measure the health of an economy. These factors are mainly computed on a quarterly and an annual basis and are indicators of the macroeconomic situation and how it has changed over the past quarter or year. You might have come across news headlines that say, “Unemployment rate dips by 10 basis points to 4% in Q2 2021.” Here, the unemployment rate is a metric, and its variation over an interval is measured by the statistic ‘basis points’.
Note: 1 basis point is equal to 1/100th of 1%. So, if the unemployment rate drops from 4.1% to 4%, it is said to have dropped by 10 basis points.
Estimation
Estimation deals with using past observations to build estimates or predictions about the future, i.e. building inferences about the future. These processes and methods to build inferences about the future fall under the broad category of Inferential Statistics. Now, it’s not just the past observations that are the basis for building estimates. There are underlying assumptions, especially when estimating economic indicators that also play a major role in building future estimates.
The second part of our sports example dealt with the chances of your favorite team winning the next game based on the observations and information available is a classic but simplistic example of Inferential Statistics. Also, you might have come across future estimates of GDP, Unemployment Rate, and a few other primary economic indicators that are reported by various investment banks and other economic research institutions and advisories. The primary ingredients behind these estimates are past observations and the assumptions made by the analyst conducting the research. You might also have noticed that these estimates vary, although slightly, with institutions. The primary reason is the difference in assumptions made by the analysts at these institutions.
This is where the idea of accuracy or error rate comes up. When you make predictions about the future, there should be a method in place that tells you are they any good? If yes, how good. An important thing to note here is that the method/model/process you use are the tools required to build estimates. They wouldn’t play a major role in determining how good the estimates are. The goodness of your estimates is highly dependent on the quality of the data and the assumptions that you make. The primary thing to be aware of when doing statistics in real life is not to get carried away by the sophistication of your methods and models.
Real-life data comes in many undesirable forms. It is large, messy, and even in some cases, incomplete. When we have a large number of observations in our data, it isn’t that easy to process the numbers by looking at them. This is when we need to take a visual approach to understand them. This is absolutely necessary if you intend to perform some advanced statistical techniques on the data. But, it is generally a good idea to look at your data as a whole. This is where statistical distributions as a tool come in handy.
There might be cases when the magnitude of data at your disposal is too high for you to process. Or the possible number of observations might just make the data collection process itself a lot more difficult, which is the case in real life. This is where using samples of observations from the total possible observations comes in handy.
SAMPLE, POPULATION, AND DISTRIBUTIONS
So, the scope of statistics isn’t limited to what we’ve seen so far. It’s much more than that. We began our quest for understanding statistics with a number of observations already recorded. Also, the sports example can be considered a case of a few observations (‘few’ here is anecdotal). The reason I mentioned the sports example to be the one with fewer observations is that all possible observations can be used to summarize or build estimates.
But, what if your maximum possible observations for a statistical experiment are on the scale of millions. Collecting all possible observations in such a case would be a challenge on all fronts ranging from cost, time, data handling, recording, and storage. With the advent of technology, handling and storage are hardly major concerns but collecting large amounts of reliable data is still a major challenge on the other fronts. This is where the ideas of ‘Sample’ and ‘Population’ come in handy.
Population
In statistical terms, Population essentially refers to the entire possible set of observations.
Sample
It is a random finite subset/s from the population used to describe it with an assumption that it represents the population.
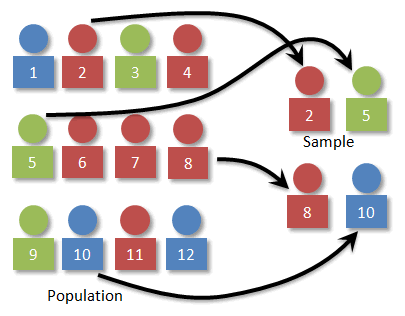
Surveys! What are they?
Surveys are the most widely used sampling techniques. They can either be standalone or part of a broader study. Respondents are given a questionnaire or a series of questionnaires. The responses recorded are observations used for further inquiry.
Say, there is a nationwide survey being conducted to find out what % of people have confidence in the country’s central government. The country’s total population is 50 million people and the body conducting the survey is willing to survey 50,000 individuals across the country.
Here, 50 million are the total possible observations i.e. Population. And, 50,000 are the observations that will be used to build a statistic i.e an insight around what % of the total population has confidence in the country’s government, which is the Sample.
The underlying assumption here is that the 50000 people surveyed are a close representation of the 50 million population. The truer the assumption, the closer the statistic would be to reality.
Thus, in order for the assumption to be as close to reality, various sampling methods are employed. The purpose is to select the individuals in the most random manner possible so as to reflect a real-life scenario.
Now, having taken all the precautions to keep the observations as random as possible, there are chances of bias finding its way into our sample, which would deviate from the survey results. This is called a Sampling error. The sampling error simply means that our sample does not represent the population.
So, can we reduce sampling error? Yes. Can we reduce it to zero? No. It is impossible to exactly replicate a real-life scenario.
How can we reduce sampling error?
Note: Detailed sampling methods are something that will be covered in follow-up articles.
- By taking a larger sample. In our example, the sample size is 0.1% of the population (50k/50M). This means we had randomly picked 0.1% of people to represent the entire population. This makes our sample too small and the chances of sampling error being large quite high. In statistics, there is a widely practiced assumption that a sample size of 10% is representative of the population.
- By having a larger number of samples that represent diverse groups within the population. This method works when you have reached an upper limit on the sample size you can consider but you have a large population size.
Distributions
Distributions are a visual way of representing your sample or the sample space if you have a set of samples. They however represent one parameter at a time. Taking our survey example, our distribution would be of the 50,000 observations.
So, how are distributions built?
The x-axis represents the range of values in your data, and the y-axis represents the number of times that value occurs in your sample space.
A few most common distributions are shown in the figure below. The most common of them is the Normal Distribution also known as the Gaussian Distribution or the Gaussian.
Although there are a large number of types of distributions other than the ones shown in the figure below, there are mainly two kinds of distributions.
- Discrete
- Continuous
Both these distributions are based on the kind of data i.e. categorical or continuous.
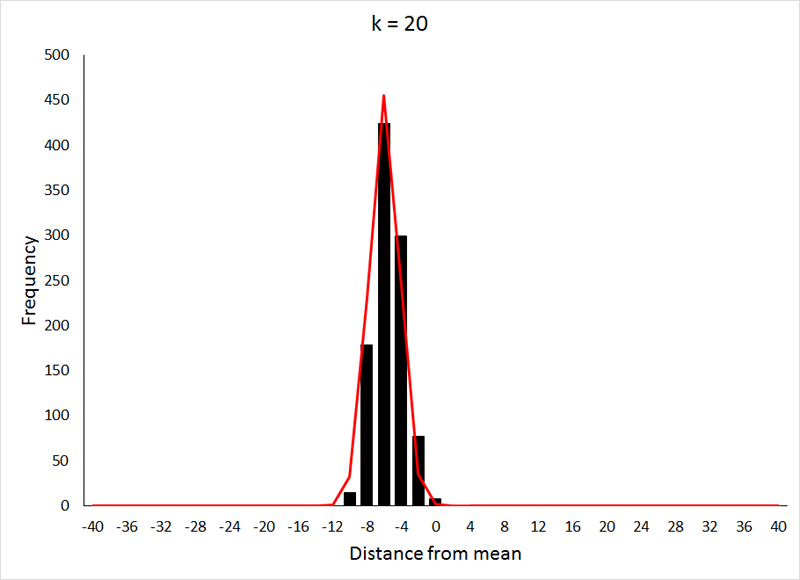
Statistics as an applied field. It can prove to be extremely helpful in making decisions. It helps build breakthrough insights, but at the same time could give misleading results when one doesn’t really know what he/she is doing. Also, the fuel that drives it, the data is an extremely important aspect. If not provided with the right data, all of your fancy statistical methods are just “Crap In, Crap Out” systems.
If you like what we do and want to know more about our community 👥 then please consider sharing, following, and joining it. It is completely FREE.
Also, don’t forget to show your love ❤️ by clapping 👏 for this article and let us know your views 💬 in the comment.
Join here: https://blogs.colearninglounge.com/join-us