Top 15 Breathtaking Applications of Data Science in Banking
Bank as an entity is something with which we are very much familiar. We don’t really need any formal introduction 😝. Adoption of technology in the banking sector is happening at a fast pace to keep pace with the competition, especially after the financial crisis in 2008. It is also very essential for banks to use technology because of the nature of business and security. Also, today it is inefficient and almost impossible for any business to make decisions that are not data-backed. And banking sector is no different.
When’s the last time you visited your local bank?
The primary function of a bank is in borrowing and lending money. We as a customer deposit our hard-earned savings to the bank. In return, they pay us annual interest. The bank plays a crucial role and uses the majority of these deposits to extend financial support in the form of loans to the businesses that want to invest and grow. Individual customer can also reach out to the bank for various types of loans which includes home loan, car loan, educational loan, etc. The customers pay an interest rate for acquiring the money via a loan. The profit margin earned from the interest rates is effectively business for banks which is the backbone of the country’s economy. All these engagements with banks i.e procuring money via a loan, using credit cards, debit cards, investment of money through various deposits, etc. comes under Retail banking.
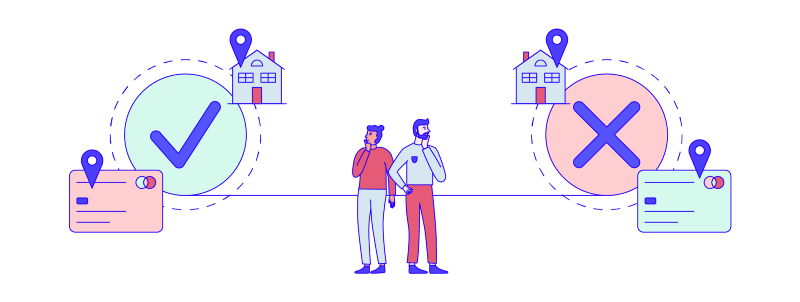
The interest in lending and borrowing business is controlled by financial regulations and highly influenced by Risk modeling to evaluate the risks associated with the financial instruments. In the past, the banks i.e. the lenders used limited data, processes, and services to evaluate the borrower profile and then come up with a decision to sanction the loan or not. Today businesses are able to achieve optimized loan allocation and pricing even if they allocate the rate of interest, tenure, and amount for a loan that is customized to the individual’s profile. With the advance in predictive data analytics, lenders can now prepare personalized loan offers for potential customers in real-time. For example, if a borrower has a poor credit history and unreliable income then the loan proposed to him is of a higher interest rate and sufficient collateral. If a borrower has an excellent credit history and a stable income then the loan proposed is of a lower interest rate. On top of that, all kinds of data — transactional data, purchase history and social media feeds are evaluated using Machine Learning for the loan sanction which in turn greatly increases their conversion rates.
In a financial institution, huge amounts of data are generated in the form of financial transactions, market price fluctuations, consumer behavior, and the country’s economic data. Credit risk modeling helps the banks to decide the probability of delinquency using Machine Learning meaning whether a person will be able to repay the loan or not. Borrowers who may have an excellent credit history and hence be considered a perfect candidate can falter and show erratic behaviors once the loan is approved. This is difficult to predict at the time of the loan approval process. Such instances can jeopardize the payment of full principal along with the interest putting the banking system and other lenders in trouble. Delinquency prediction models make use of the borrower’s past loan data, and transactional history, when the loan EMIs were not paid in full, repayment history along with missed due dates helps in averting this credit risk. It helps in deciding renewals of credit lines and ascertaining if the customer can stick to their repayment schedule. Thus, by using the various data sources the banks can significantly lower their risk and take corrective actions. Along with the usual transactional data, income, demographics, etc social media data can also be leveraged in building models to understand customer behaviors. It gives a deeper insight into a borrower’s life for example — a major life event such as a divorce or the birth of a new child can lead to different expenses and transactional patterns. This can help in resetting the repayment deadlines as well as tracking the user behavior more closely to mitigate credit risk.
Types of Credit Scoring
Application Scoring
The first uses customer data from the time of the application as well as information about the loan to predict whether the customer will be granted the loan or not.
Behavior Scoring
Once the loan is granted, the banking system keeps track of the user behavior i.e. income and expenses. Survival analysis models will take into consideration the user behavior and will predict whether a borrower will default, exactly when and how long. It helps prepare for imminent conditions, more accurately predicting possible losses and allowing better use of funds.
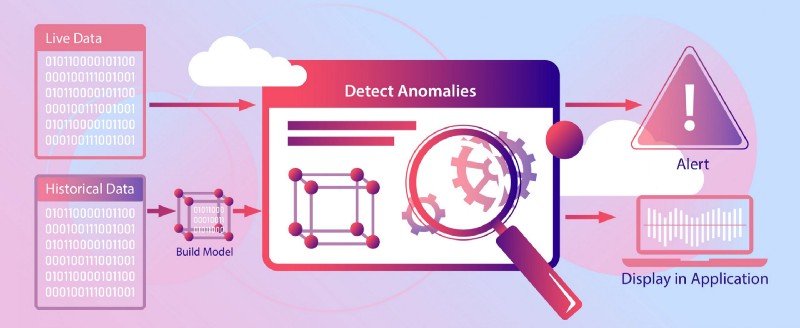
We may often come across instances where there would be frauds occurring within the banking space, be it payment fraud, credit card fraud, or fraud happening through other sources as well like fraudulent/speculative trading, rogue trading, and regulatory breaches. The digitization movement is making the process easier but also accompanies a huge number of fraudsters along with it.
Banks operating in India reported fraud of Rs 49,2000 crore as on March 31, 2021.
Machine Learning models enable the detection of anomalies in payment frauds where there might be any kind of deviations from the normal transactions. Thanks to numerous transactional data. The ML-based models track the daily user behavior and flag instances where there is a higher fraud likelihood. Credit card fraud is one of the most common fraud instances but this is being reduced by verifying the PAN Card, Aadhar card, mobile data usage, social media usage, etc.
Investment Risk Modeling helps early detection of risk, predicting the likelihood and magnitude of the impact of unfavorable events on the financial outcomes for any business entity, portfolio, or individual. Financial analysts will attempt to model risk as a means of comparing the attractiveness of different investment opportunities.
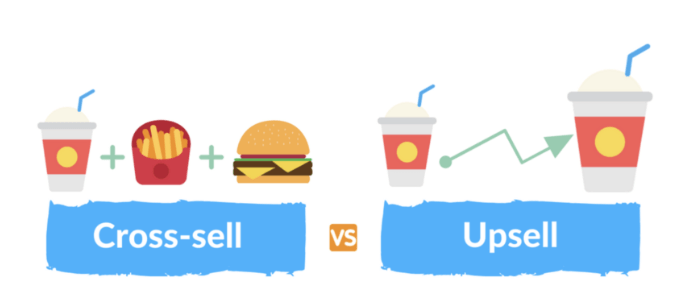
Other than managing risk and increasing profits. Banks use Data Science in selling new products to existing customers and acquiring new potential customers. Banks regularly collect customer data(often unstructured) data from social media to assess how customers view the brand and its offerings which in turn helps in launching marketing campaigns with an effective customer segmentation strategy. ML-based models help in Cross-selling and upselling in allow better customer experiences by identifying the customer needs. For example, a customer who is holidaying at an overseas location will get a push notification for the best foreign exchange deals. Recommendations for similar financial products can be made with the help of recommendation engines. For example, if a customer looks at Amazon products and pushes items to his cart, he could be given a recommendation for the Amazon ICICI Bank credit card offer as it will help the customer with additional benefits of cashback as well as the sale of the card for the bank. These data-driven insights are helping the banks in providing personalized services to the customers.
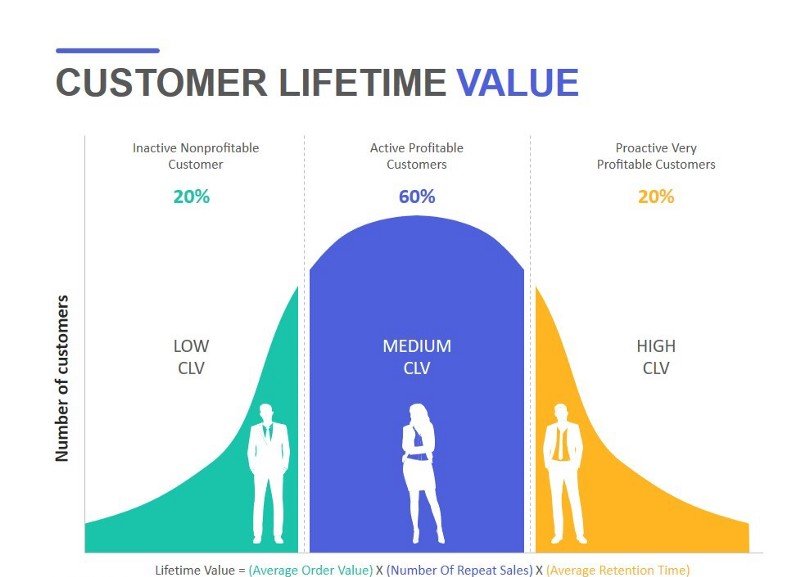
The world of business has completely changed and revolves around its customers more than ever. The customer-centric approach is the new norm in today’s market. The reason for that is the customer have plenty of alternative options at their fingertips hence banks need to implement emerging technology-based solutions to retain their customer or increase their brand loyalty. Hence the cost of retaining existing customers(preventing customer churn by offering discounts, cashback, customized offers, etc.) is as costly as acquiring new customers. It is these tradeoffs that the banks are supposed to deal with. Customer lifetime value prediction is one such use case that allows the banks to make a prediction about the revenue a customer will generate through purchases/transactions over a lifetime. This ML model helps the banks identify which customers are high-value generating customers and will make efforts to retain them. This helps in targeting the right customers rather than trying to retain all the customers.
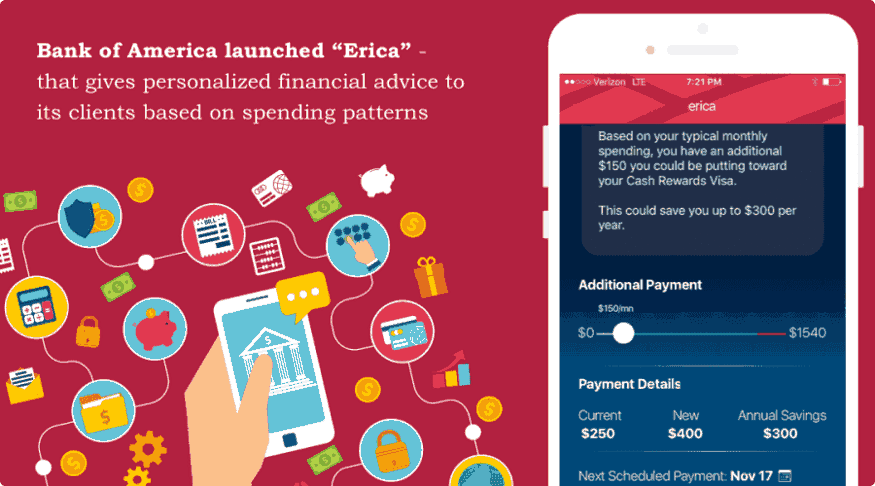
To stay in the competition, Financial institutes have to rethink their customer experience strategy and be prepared for rising customers’ expectations. As customer support is a crucial part of brand and business, Conversational banking is redefining the experience with help of text, voice, and rich formats. Chatbots(Virtual Assistants) and Conversational IVR have several benefits to businesses and customers. Especially, customers prefer a chat-first experience and need an instant answer to their queries without waiting for a human on IVR. Businesses on the other hand are benefitted from cost reductions as they do not need to maintain huge call centers with manpower. Chatbots are also able to provide a vast depth of insights into customer behavior, frequent queries, statistics, and many other things. For example, Bank of America has created Erica which is an AI chatbot. Erica helps a customer maintain their finances at Bank of America. It helps you keep a track of your financial transactions, provides personalized services, and also gives predictive credit scores. These AI chatbots are revolutionizing the customer support wing of the banking industry.
Conclusion
To gain a competitive edge, banks must not ignore the crucial significance of data science, integrate it into their decision-making process, and develop strategies based on the actionable insights from their client’s data.
Data Science is rapidly evolving and its capability to deploy ML models to real data, getting more and more data-driven accurate results.
If you like what we do and want to know more about our community 👥 , please consider subscribing, following, and joining it. It is completely FREE.
Also, don’t forget to show your love ❤️ by clapping 👏 for this article and letting us know your comments.
Join here: https://linktr.ee/colearninglounge