Top 7 Breathtaking Applications of Data Science in Finance
Finance is one of the most crucial sectors for the world and the country’s economy. Although the use of a data-driven statistical approach to making decisions was already part of the business, recently the advancement in the Data Science field and the shift from paper to a paperless financial transaction created humongous data points to analyze the behavior of spending and investing. JPMorgan’s machine learning-based COiN platform reviews 12,000 annual commercial loan agreements in just a few hours, as opposed to the 3,60,000 manual man-hours. The benefits of applying data science in finance are diverse. And this is just one example.
Today we prefer paying digitally even if it is 1 Rs, Isn’t it?
Before we move further, let’s try to understand “What Finance is?”
Money, currency, investment, asset, and risk all these words are related to finance. We know that already. But the question is, what exactly is Finance?
Managing money is finance. It can be done by a 10-year-old kid, a CFO, or a finance minister.
The goal is simple, to get the most value out of it. Next question, what is money and how is it different from the currency?
Money is a portable, durable, divisible, interchangeable medium of exchange of value which is a unit of account and stores a value — Aristotle
If you remove ‘stores a value’, it becomes currency.
Gold, silver, and bitcoin are money because of their physical/digital scarce nature which gives them value. Rupees, dollars, yen, etc do not have their own value.
Basically, finance goes around money, which increases in the form of assets like stocks, gold, and bitcoin and there are systems that help individuals and organizations manage them. Most of us know or are dealing with stocks, “Buy when you see a dip and sell when there is a rise” is the very first and most common advice we have got. But definitely, it is not that simple, as prices of the stock are so volatile, by the time you try to figure out what is happening and past performance, you’ll see your fort turning into ashes.
The first Covid-19 confirmed case in Indonesia is on 2 March 2020. After that, the composite stock price index has plunged 28% since the start of the year and the share prices of cigarette producers and banks in the midst of the corona pandemic reached their lowest value on March 24, 2020.
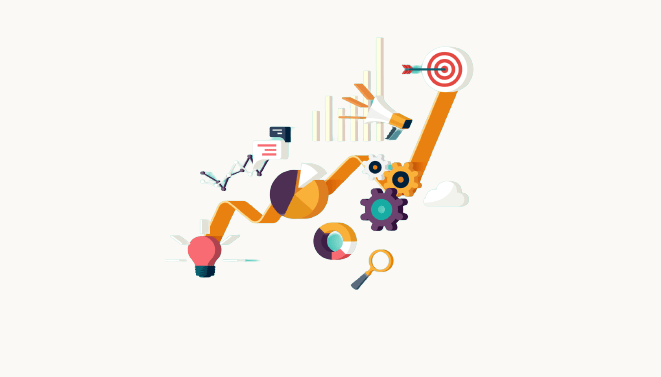
Generally, everyday experts try to predict asset prices, be it a house, gold, or even stocks. As it is simply not limited to supply and demand but rather complex and challenging because of its dynamic nature which makes Asset Price prediction a crucial use case of Data Science in Finance. To do that two complementary approaches are used: Technical analysis and Fundamental analysis. While technical analysis solely looks at historical prices, the fundamental analysis consists of understanding the external and internal factors that influence the price of a certain asset.
The changing trend of the stock price has always been identified as a very important event in the economic field. As stock prices are affected by various internal and external factors, such as domestic and foreign economic environment, social/political situation, industry prospects, financial data of listed companies, stock market operation, etc. Thus, the forecasting method also has a different emphasis. Based on Statistics, Probability Theory, and Deep Learning, a time-series linear forecasting model to predict the short-term stock price with a large amount of long-term data.
Traditionally we had few assets like land, currency, and metal. But now we are in the age of NFT and if we don’t talk about digital currency, then we would be nothing but a negative outlier.
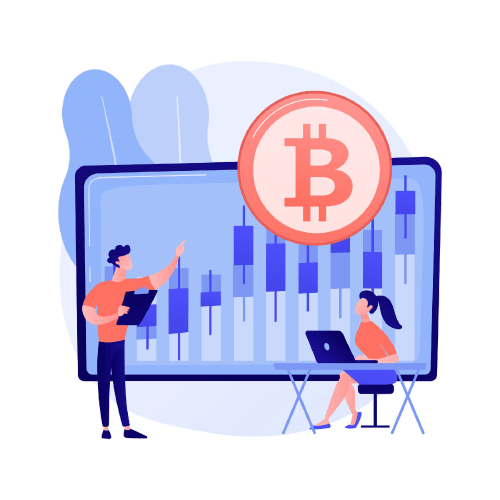
Cryptocurrency is the latest (digital) asset in class. It has value because of its digital scarce nature. It’s limited, like Gold because we can not print or mine it forever. Bitcoin investment strategy is the latest use case and one of the most talked-about investment assets of the year. A few years back few big banks said, cryptocurrency is a bubble and those same banks are working on their own cryptocurrency.
Remember when Elon musk changed his bio to ‘Bitcoin’ and all those ‘to the moon’ trends, changed everything for good. In a couple of months, bitcoin went from 30k to 65k and it seems it actually reached the moon, and then it settled. Obviously, No one could have predicted what happened back then. It was not just Elon’s tweet but people’s sentiment is involved whenever the market sees this kind of disaster. Because of its high volatility, it can be a profitable investment. Data Science can be helped to find the patterns of the dynamically changing crypto market by processing vast amounts of data.
The reason bitcoin or any cryptocurrency is volatile is that our market does have an infrastructure to support these kinds of anonymous transactions. Hence it is very difficult to predict its price with sentimental analysis of the market.
General strategies used in the crypto market are HODL(Hold on for dear life). Another strategy is Hedging and Automated Cryptocurrency Trading where Data Science is a big help to make safe decisions.
What is Hedging?
For example, the Bitcoin price predictor shows that the price might fall tomorrow. So, you sold your 1 bitcoin at 50k and it happened. Bitcoin falls to 40k. Now you bought that coin back at that price. So, you still have a coin and you are 10k profit.
Otherway is to trade this into other coins. You can sell your bitcoin and buy a few Ethereum and DOGEcoin because you can see a surge in its pricing. You follow the trend, make some profit and when you see a decline in trend, you sell those coins, buy bitcoin, and still have profit.
Now we know that assets can be managed, invested, and valued by individuals also. But sometimes it’s more than people. It can be the government or a big firm that is trying to build capital by following tactical investment strategies which lead to the importance of investment banking.
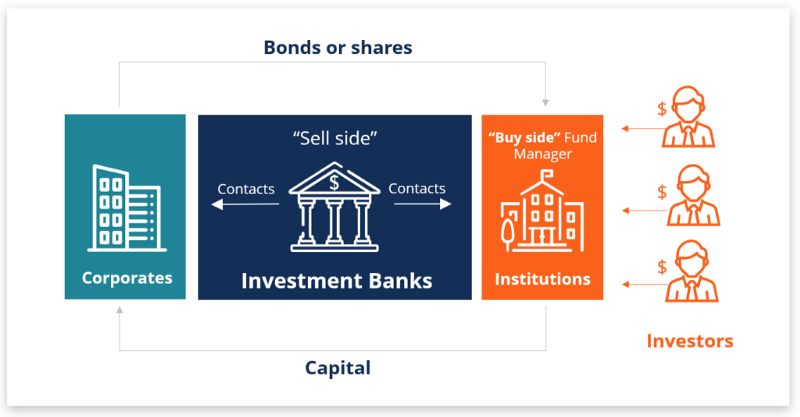
JPMorgan Chase, Goldman Sachs, Morgan Stanley, and Barclays are all of these fancy and giant institutions that are Investment banks. They offer almost the same services as the retail banks but on a greater scale at a bigger risk to other institutions, governments, or wealthy individuals. Their main revenue source is advisory. They give recommendations to institutions on how and where to invest money, how and predict when to raise capital, through debt or equity, how to go for IPO, offer services to create a market for an institution where they can meet potential buyers, help institutions build a strategy around mergers and acquisitions and many more. It is done with the help of different ML and Time Series models to find optimized prices, fraud detection, market segmentation, and sentiment analysis to retain or acquire customers.
In investment banks, this role is called “Quant” (Quantitative analyst). A quantitative analyst or “quant” is a specialist who applies mathematical and statistical methods to financial and risk management problems. S/he develops and implements complex models for firms to make financial and business decisions such as investments, pricing, and so on.
When a 10-year-old kid starts managing his pocket money, grows himself into a position where he manages different kinds of money/currencies at his fingertips, and that too for multiple people, institutions is called Portfolio management.
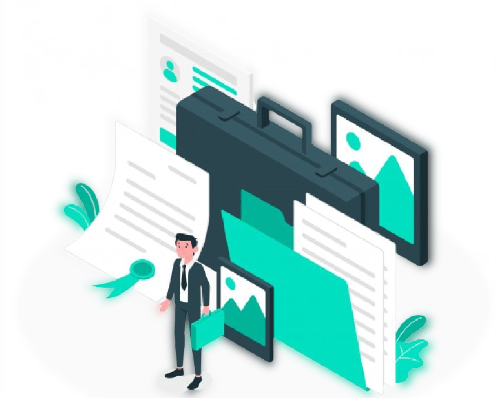
Portfolio management is all about creating/working an investment and risk strategy to meet an objective by doing asset allocation for some individuals or institutions. Strategy to invest that asset in stocks, bonds, gold, bitcoin, NFTs, or more and understand the risk to produce a maximum value. The traditional or manual techniques of collation and human analyst interpretation simply prove to be ineffective or do not scale for maximizing profit and minimizing loss. Data science can help these managers analyze fundamental and alternative data sets to identify new investment opportunities.
Ultimately, it is the combination of data, technology, and human talent(Financial Analyst, Risk Analyst, Quantitative researcher, and more) that leads to the successful incorporation of these techniques into an asset manager’s strategies.
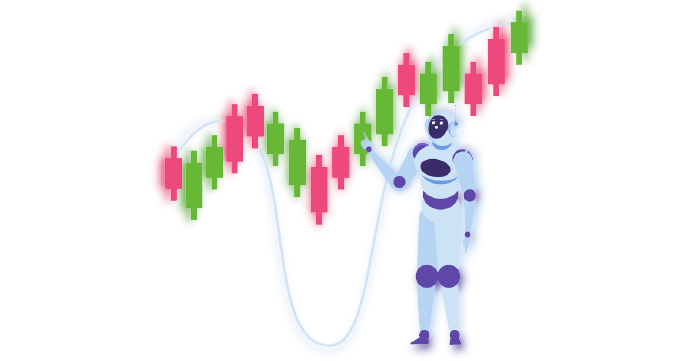
We must have seen movies based on the stock exchange, where brokers are shouting to buy and sell a stock. That can be a manual approach to trade. Things have changed. Hundreds of companies emerge every year. It’s humanly impossible to keep track of names, trading in the stock exchange hence in Algorithmic Trading with the help of Data Science we can consume real-time data from unstructured and structured sources to find trends and patterns that might otherwise have been hidden and correlate that with the historical performance of that asset to make buying/selling decision.
Algorithmic Trading is the process of using computer programs to follow a defined set of instructions for placing a trade to generate profits at a speed and frequency that is impossible for a human trader. It also uses the power of real-time analytics and makes trading more systematic by ruling out emotional human impacts on trading activities. It also improves these odds by using recommendation algorithms to make better decisions.
So far we have seen “Portfolio Management” which is creating an investment strategy and “algorithmic trading” which is automating the whole trading process. So, What if we combine both of these? Means, we remove portfolio managers out of portfolio management and replace that with an automatic and algorithm-driven financial platform that understands customers’ goals, and current financial situations and uses that data to offer advice to invest customers’ assets automatically.
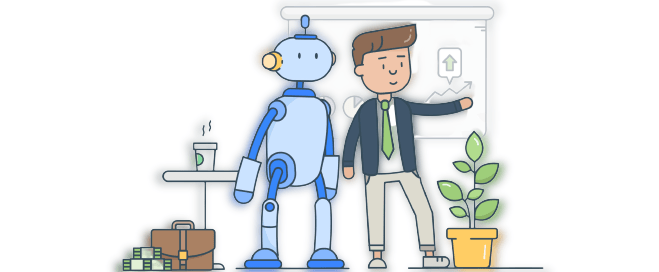
Robo advisors are a potential solution to the complexities of financial decision-making — Jill E. Fisch, a law professor at the University of Pennsylvania at a conference of the Pension Research Council.
Portfolio managers or human advisors can be costly and inefficient at a time. As it might take considerable effort and expertise to consider changing market trends. Also, it needs deep trust and comfort to work with a human advisor on financial matters.
On the other hand, Robo-advisors can consolidate clients’ data like their financial objectives, risk appetite, and time spans, with the right resource distribution that qualifies clients’ necessities. While making this decision, they utilize numerous calculations including AI models to make the best fit for the client. In this process, they take lots of actions into consideration as well such as rebalancing the portfolio or performing tax-loss harvesting. This in turn increases profitability while making judgments at the right time for the portfolio. But the system needs to understand that money is a very emotional part of everyone’s life. When we are making a long-term plan, we make them based on logical and emotional decisions. A human advisor can understand emotions, can make a personalized plan,s and challenge why a user is not taking risks even when numbers are in favor, Robo can’t.
Almost every use case in Finance must have been done manually by analysts but the future is different. Humans can’t have the accuracy of a machine. We can’t correlate numerous parameters, a machine can. We can’t study different data sets at a time but a machine can. Instead, we can teach the machine to learn from data and make accurate predictions to assist decision-makers to make critical and unbiased decisions. As data science matures and analytical infrastructure arrives out-of-the-box via SaaS, we’ll see this accelerate.
If you like what we do and want to know more about our community 👥 then please consider sharing, following, and joining it. It is completely FREE.
Also, don’t forget to show your love ❤️ by clapping 👏 for this article and let us know your views 💬 in the comment.
Join here: https://blogs.colearninglounge.com/join-us